
Image-based Modelling for Electric Vehicle Charging Networks
ICM/MCM Contest, 2018, in collaboration with Lily Li and Gavin Ding
Abstracts.
Introducing EVs (Electric Vehicles) to our daily life can significantly alleviate the worsening environmental conditions and give a feasible solution to global energy crisis, bringing us a more sustainable earth.
However, one of the inextricable problem of promoting EVs is the construction of charging network due to their longer charging time and higher charging frequency. So optimizing the charging station system would be crucial, especially in congested areas.
The properties of charging stations under determination are their quantity, placement and intra-station configurations and those could dramatically change in different regions. To diversify our model, we chose the US, Ireland and Singapore. For each country selected, we build more than one model to accomplish the scheme.
Basically, our team started from determining the car population density. For the sake of unreachable data, we applied night-light map to find a correlation of its brightness and car population density. Once the correlation established, the pixel-wise brightness data of night-light map were conducted for the hierarchy of lands and further calculation of EV charging demand in a certain area of land. The lands of a country are divided into 4 categories: urban, suburban, rural and unpopulated by its brightness. Simultaneously, because of the convenience for both designers and builders, a fixed configuration (about superchargers/normal destination chargers) in stations of each category of land is suggested. Consequently, the quantity of stations can be determined by the demand and station capacity.
Afterwards, the placement of stations was achieved with SLIC superpixel clustering, which aims to cluster colored points with higher similarity of color and proximity. We logically converted a part of traffic congestion map of each category to an image with color nodes. Then by SLIC clustering, the estimated service area and placement of station will be reachable.
In case of Ireland, chronological factors have to be considered. So gray forecasting model GM (1,1) and species competition growth model were utilized respectively for predicting the car population growth and the proportion of EVs in the coming years, with static analysis while 10,30,50 percent of cars become EVs.
Additionally, we involved the factors of government interventions and innovations that will promote or replace EVs in the future, which also showed obvious effects.
Finally, a handout for leaders of countries who are attending an international energy summit to refer was extracted from our consequences and expected to make a difference on global energy-saving.
Backgrounds.
Due to the increasing effect of air pollution and fossil fuel shortage on the everyday life, people have to compromise on their ways of transportation. Strictly speaking, the major attempt is to swap their fuel vehicles to electric vehicles (EVs). As EVs mostly run on electricity, the emission and fuel consumption would be significantly reduced, which will be an obvious compensation to our environment.
Driving EVs (not hybrids) seems rare nowadays, but when it becomes a policy of multiple countries with a ban of conventional fuel cars in less than 30 years, everyone would be compelled to do a swap on his/her cars.
Therefore, the downside of EVs should be considered then: for recharging them is a noticeably prolonged process and for their fuel (battery) capacity seem less than conventional fuel tanks, finding convenient charging stations will certainly be mandatory for all drivers. In turn, construction of the infrastructure--- charging stations and networks could be massive and complicated.
Consequently, as a team of ICM, we are supposed to do some work of modelling towards determining the distribution scheme---intra-station configuration, quantity, and placement of charging stations.
Submitted Paper.
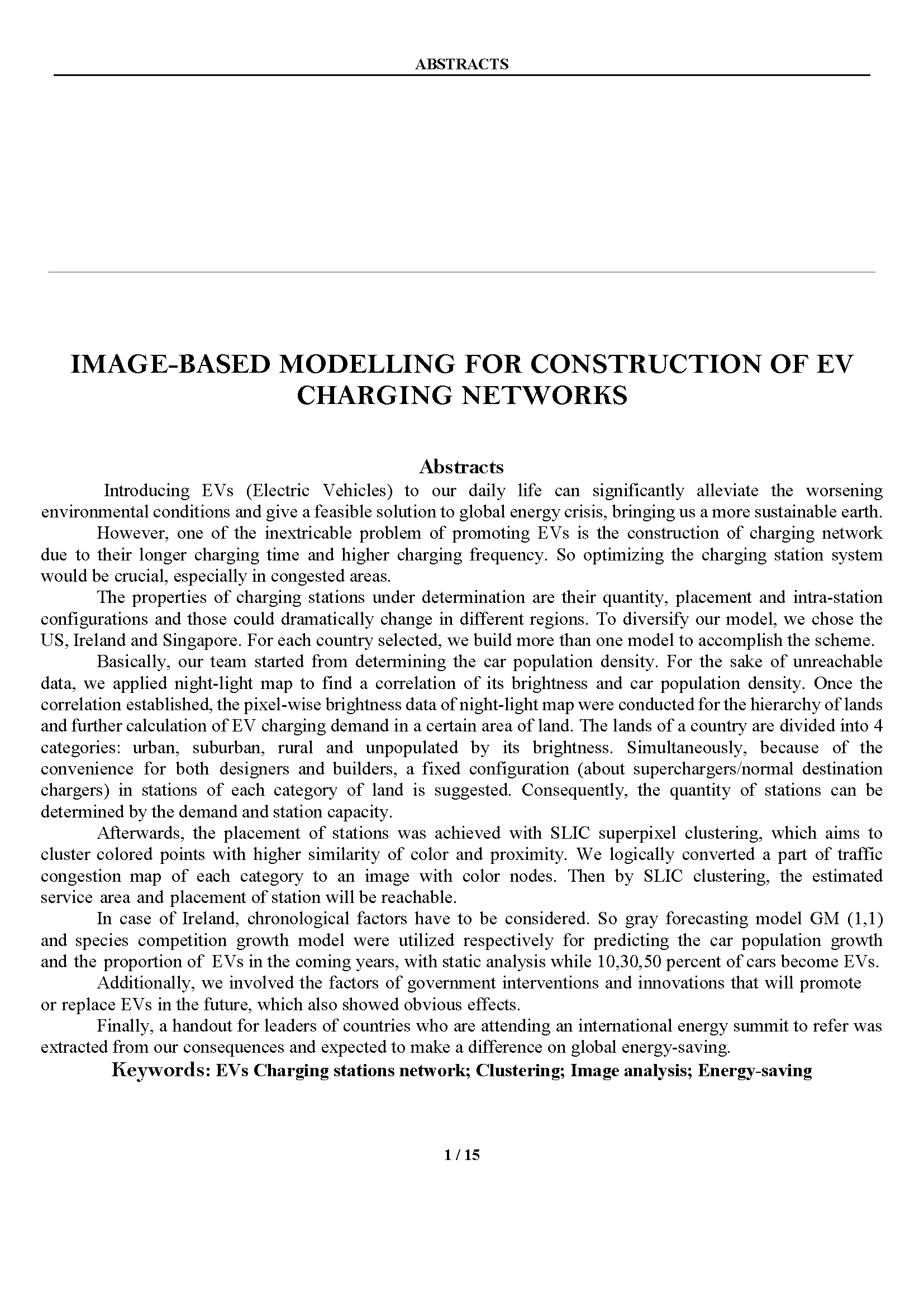
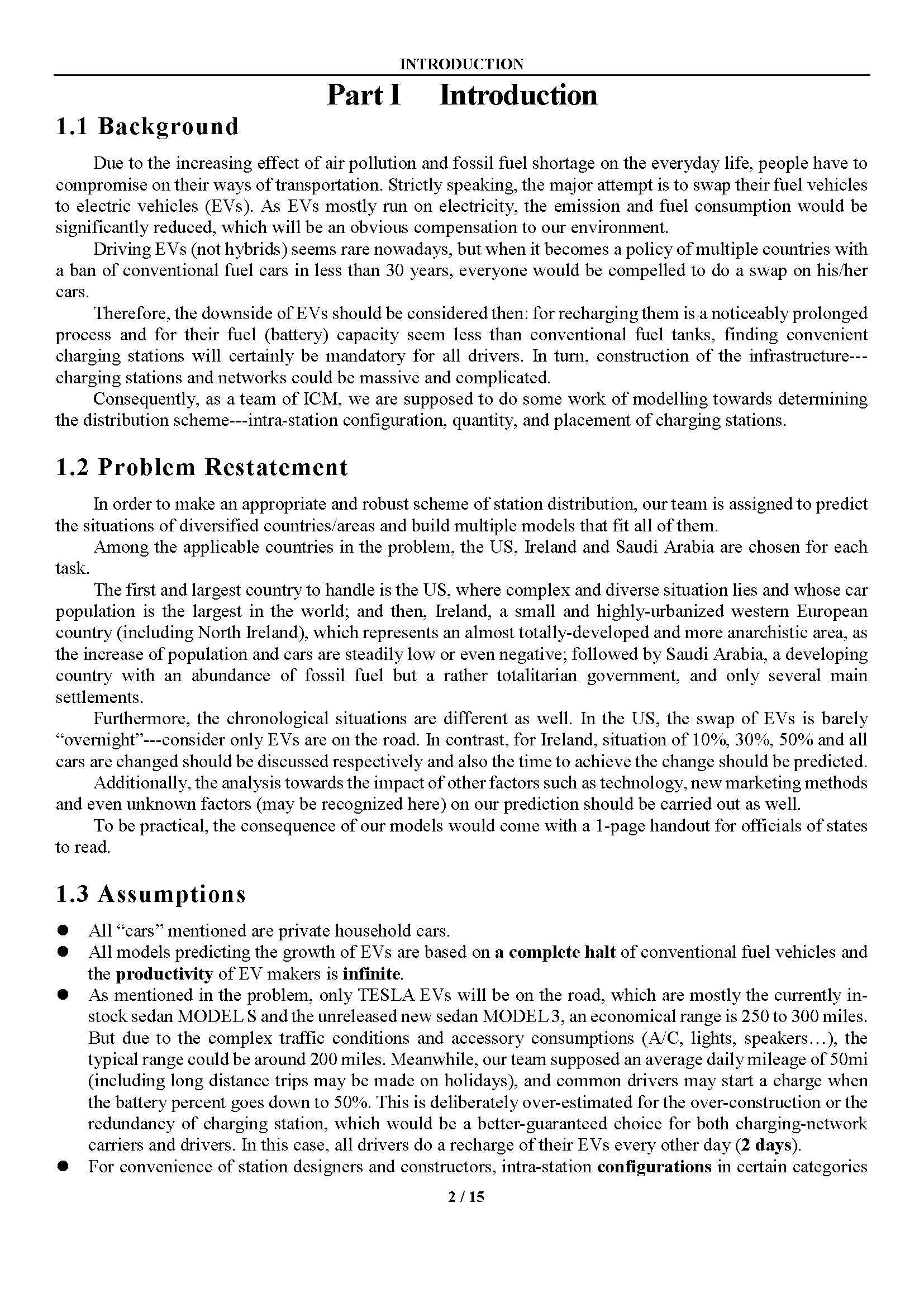
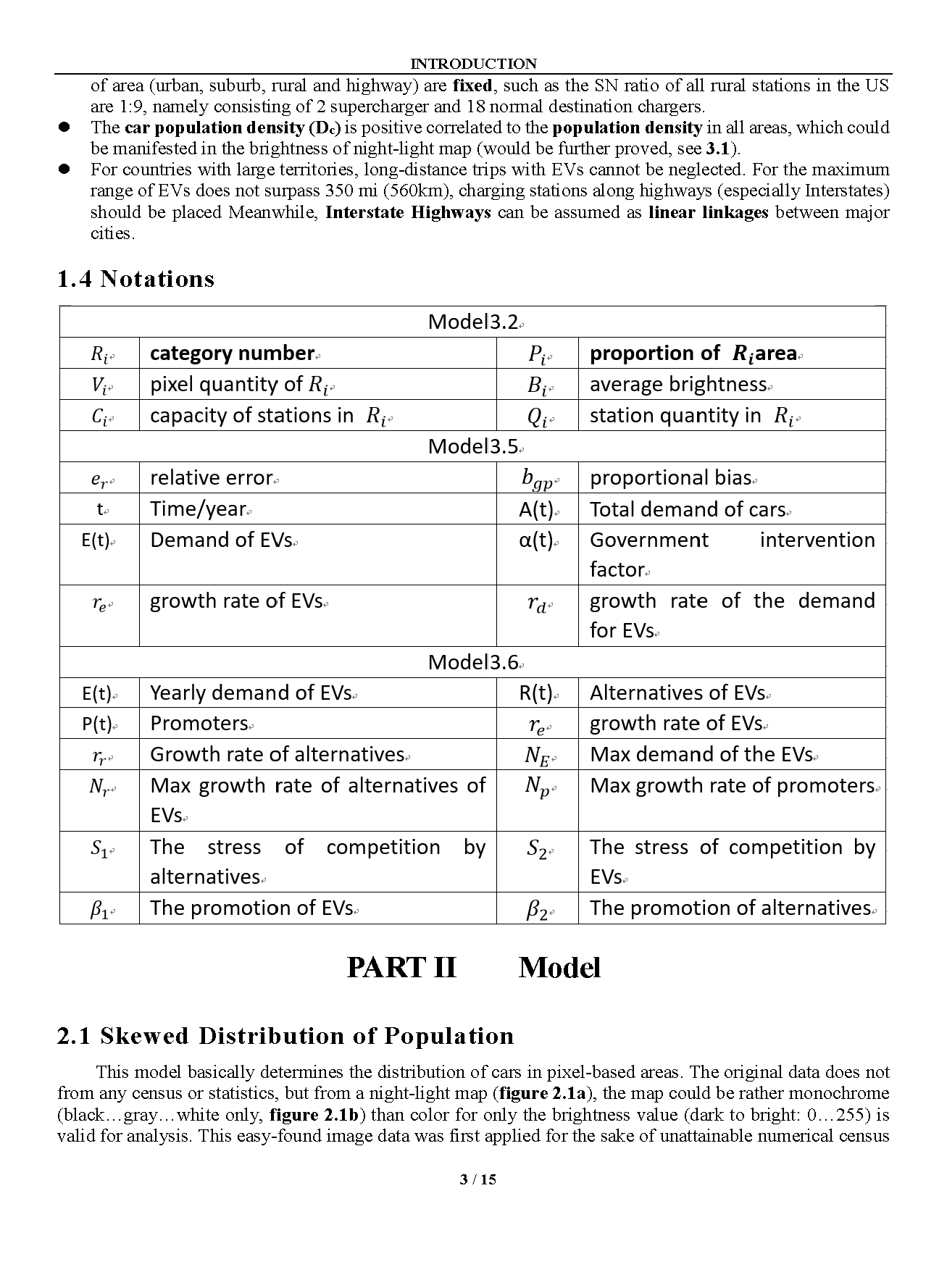
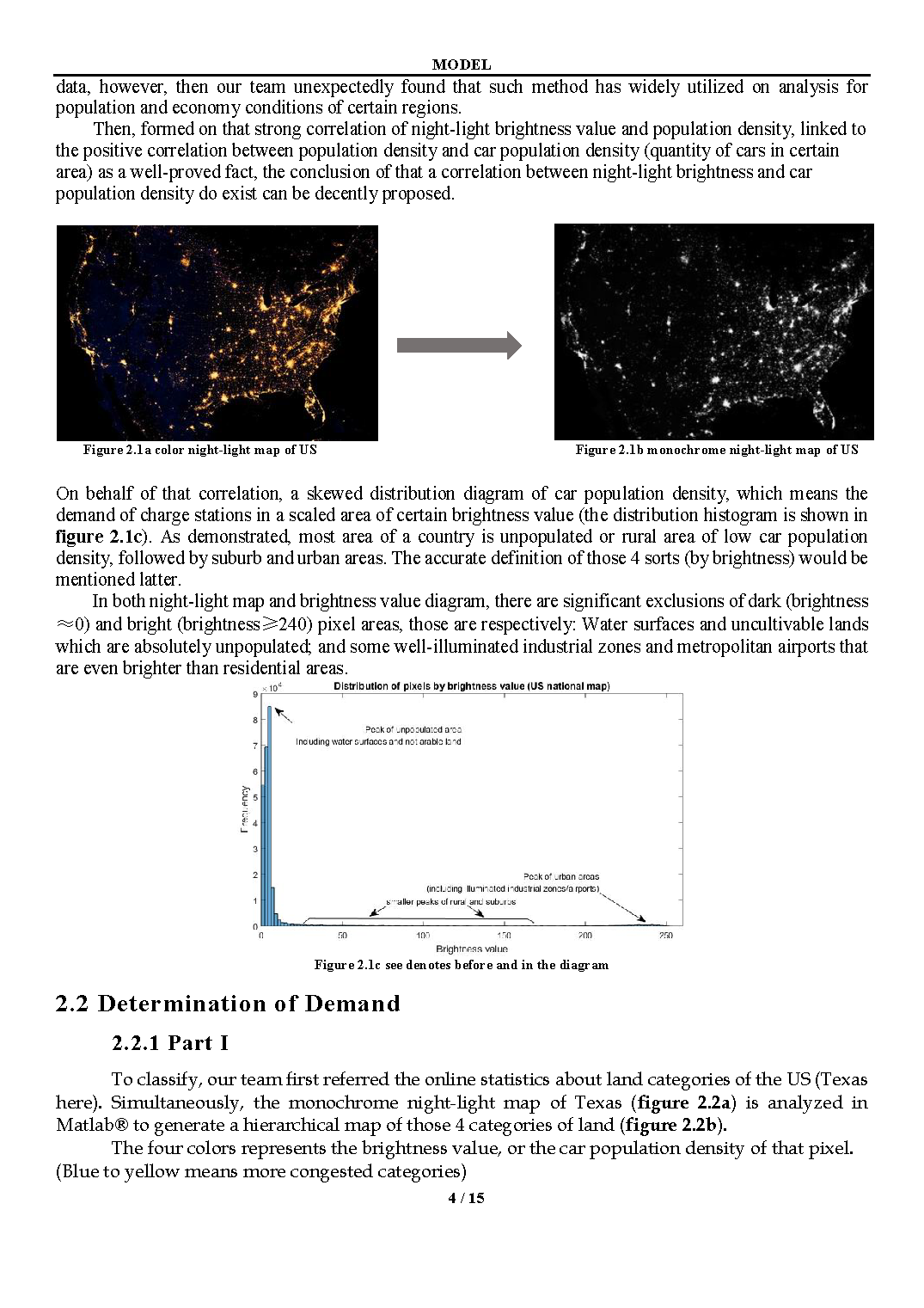
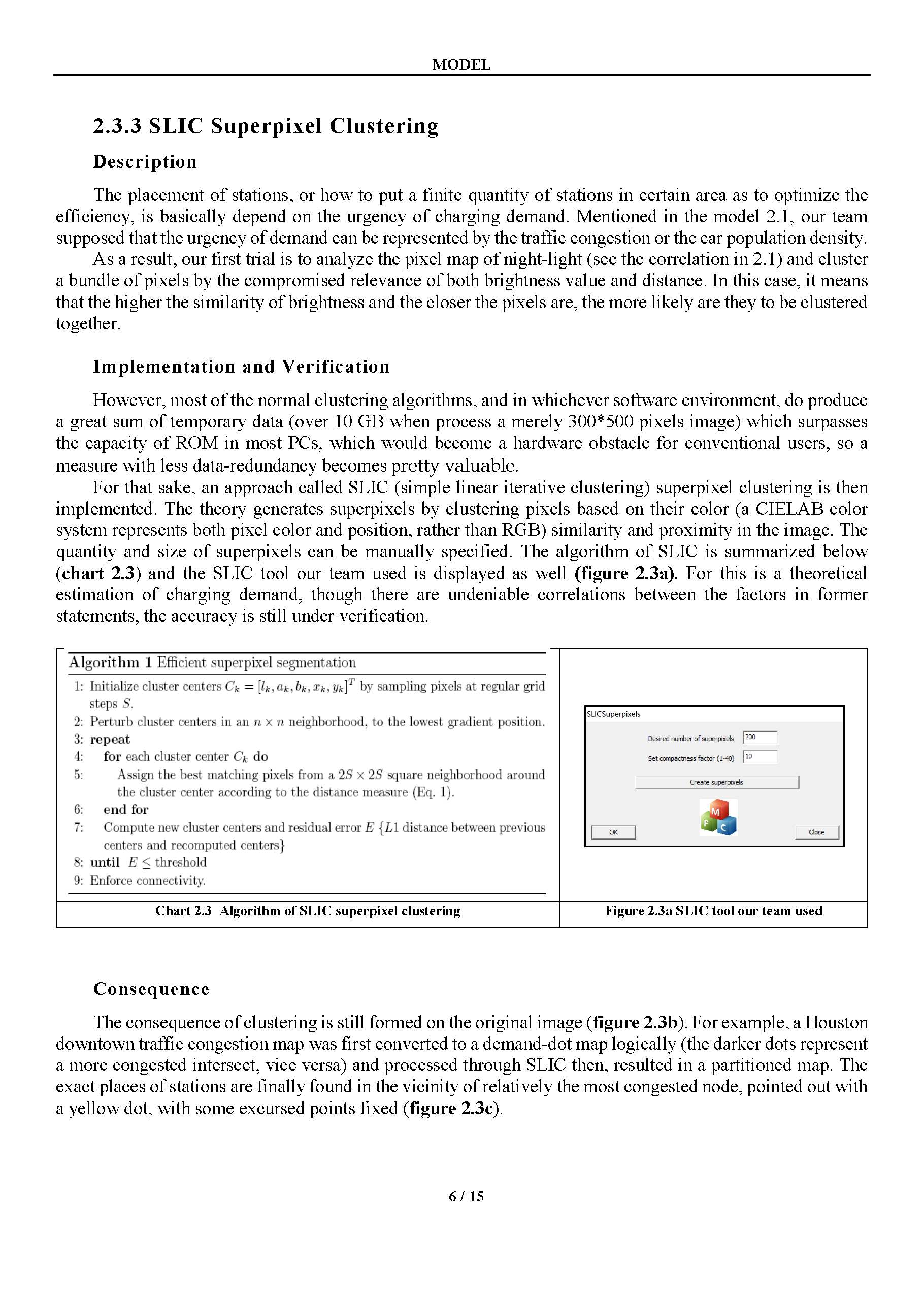
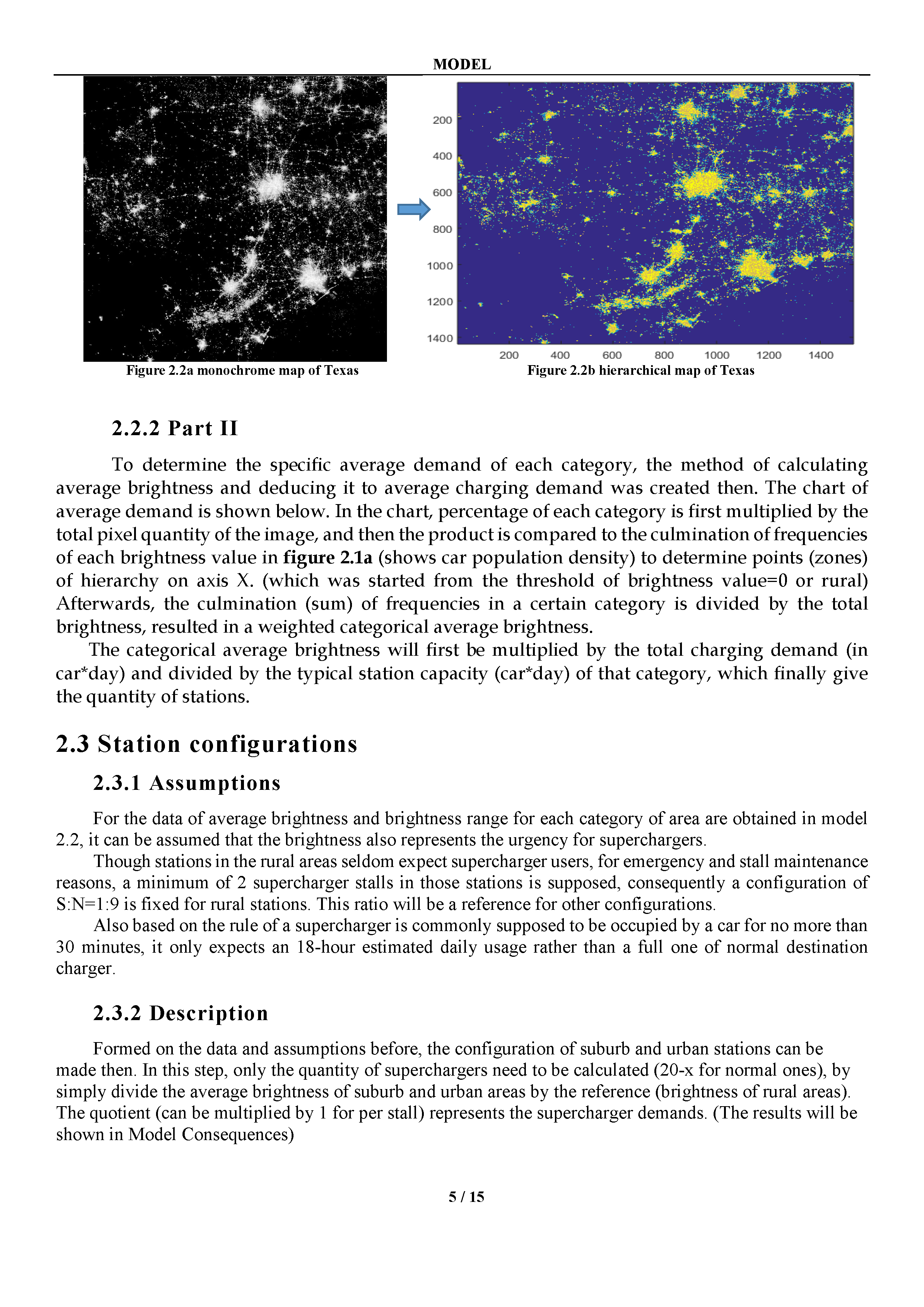
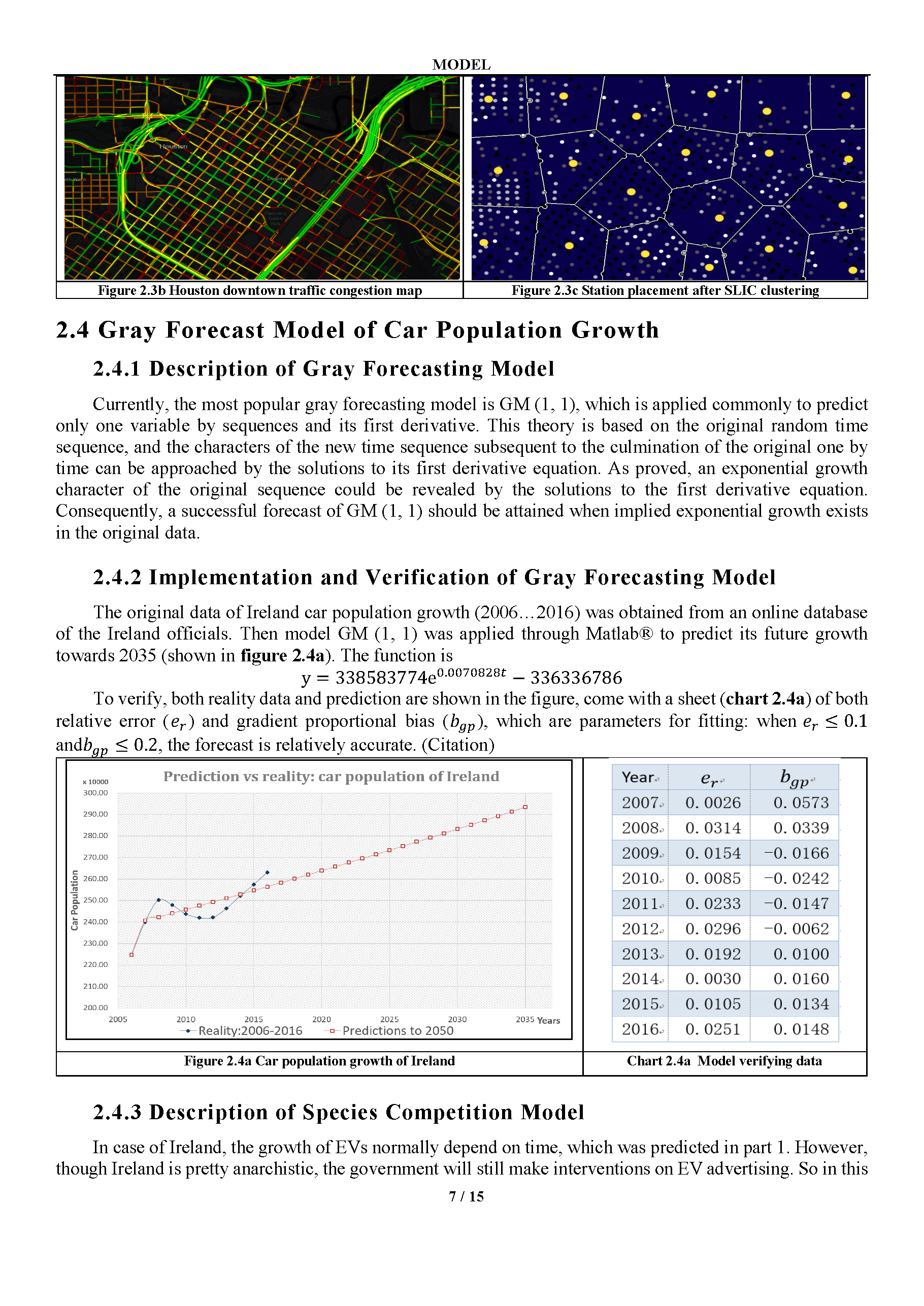
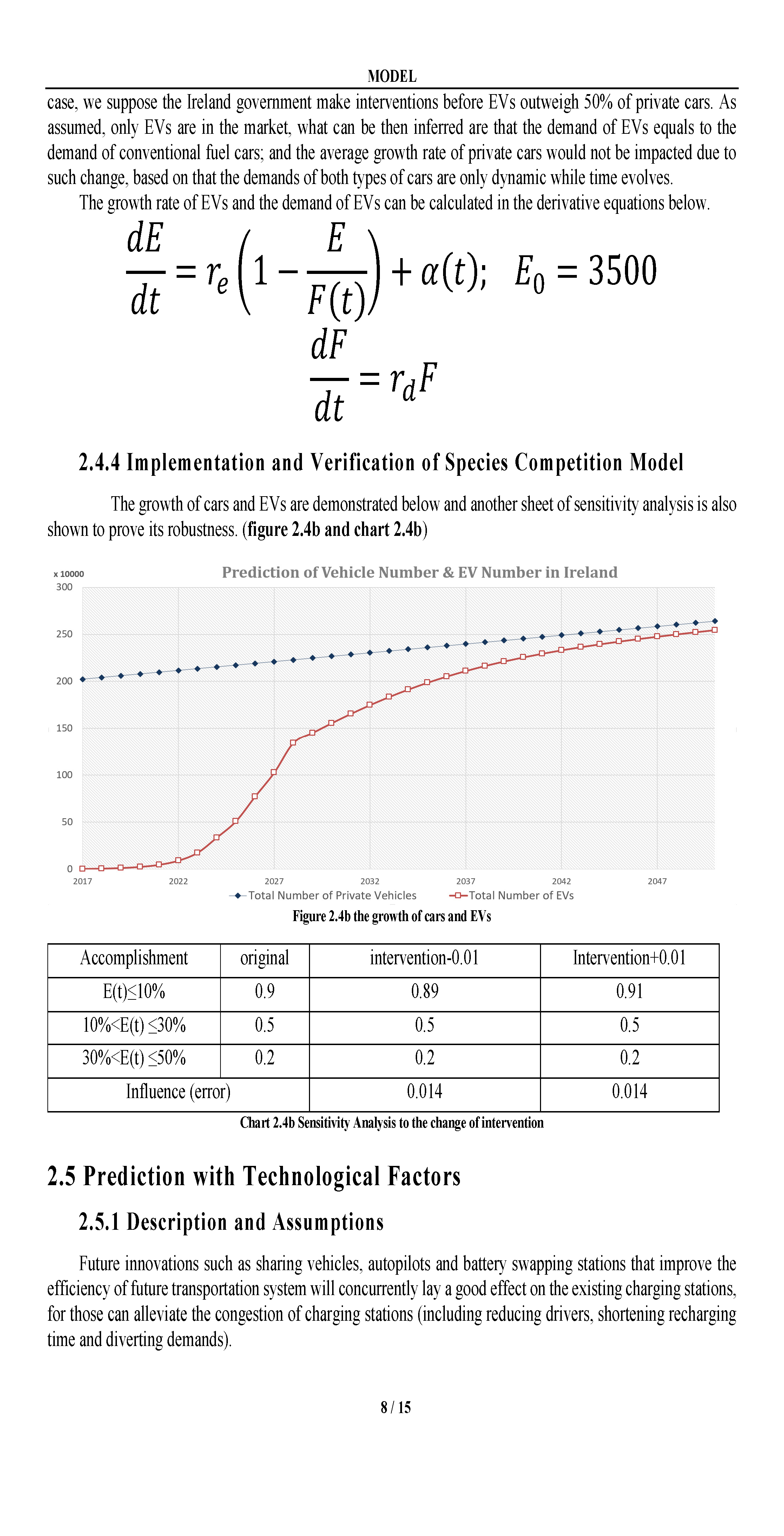
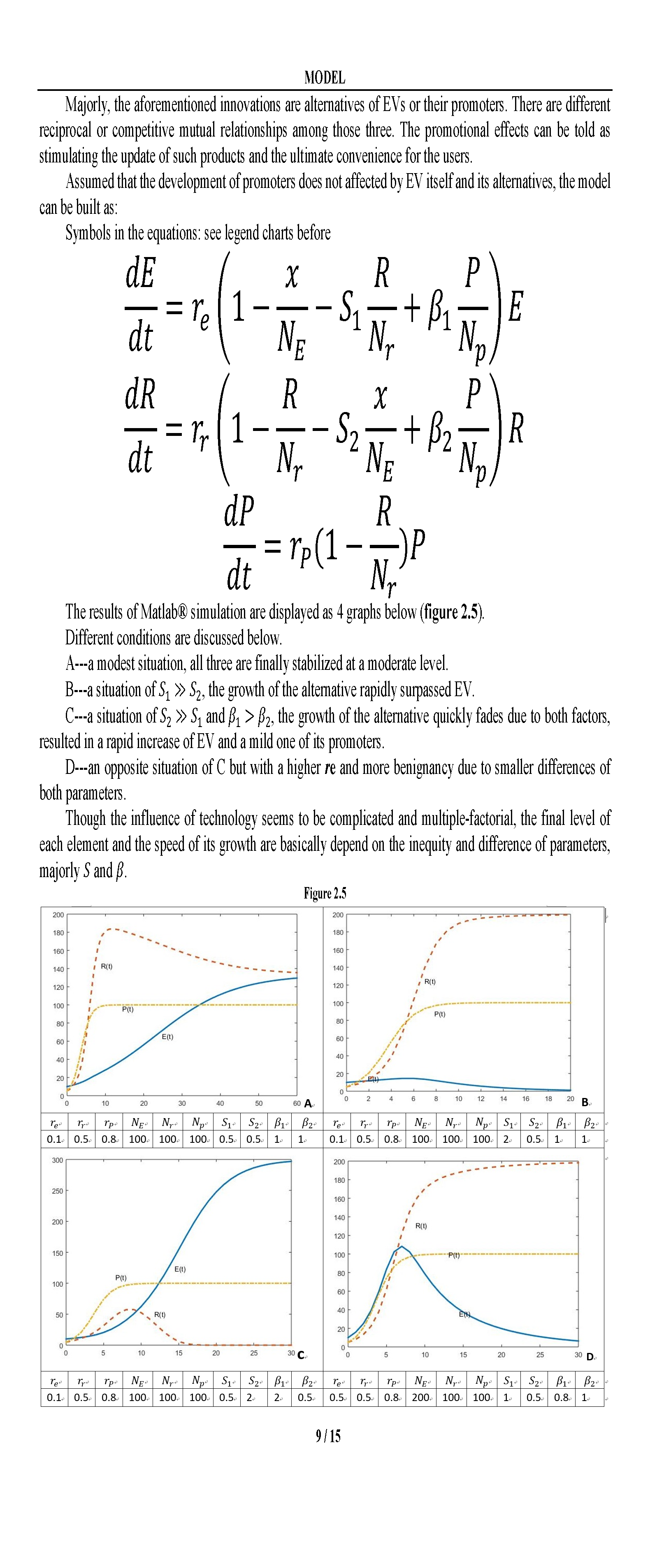
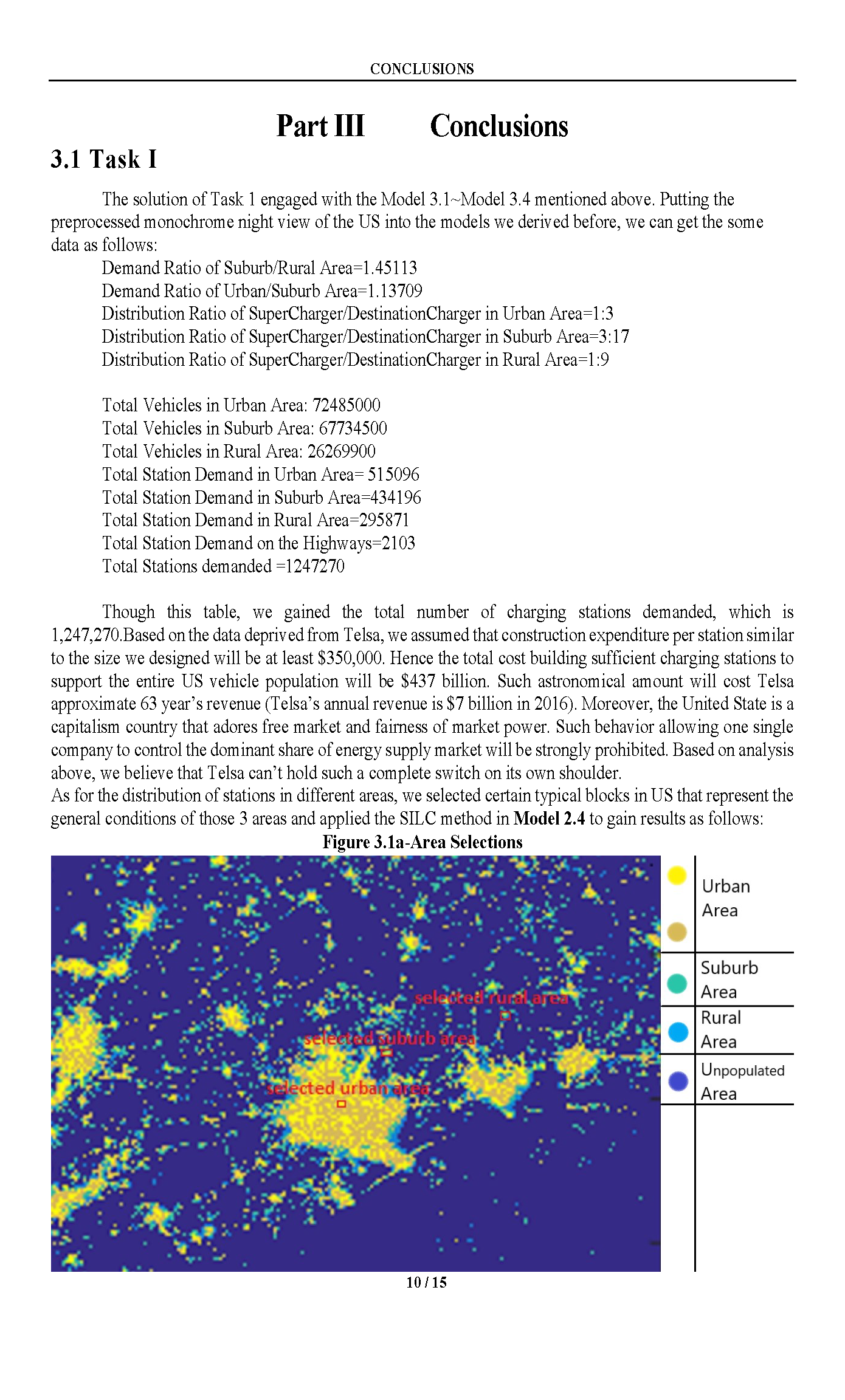
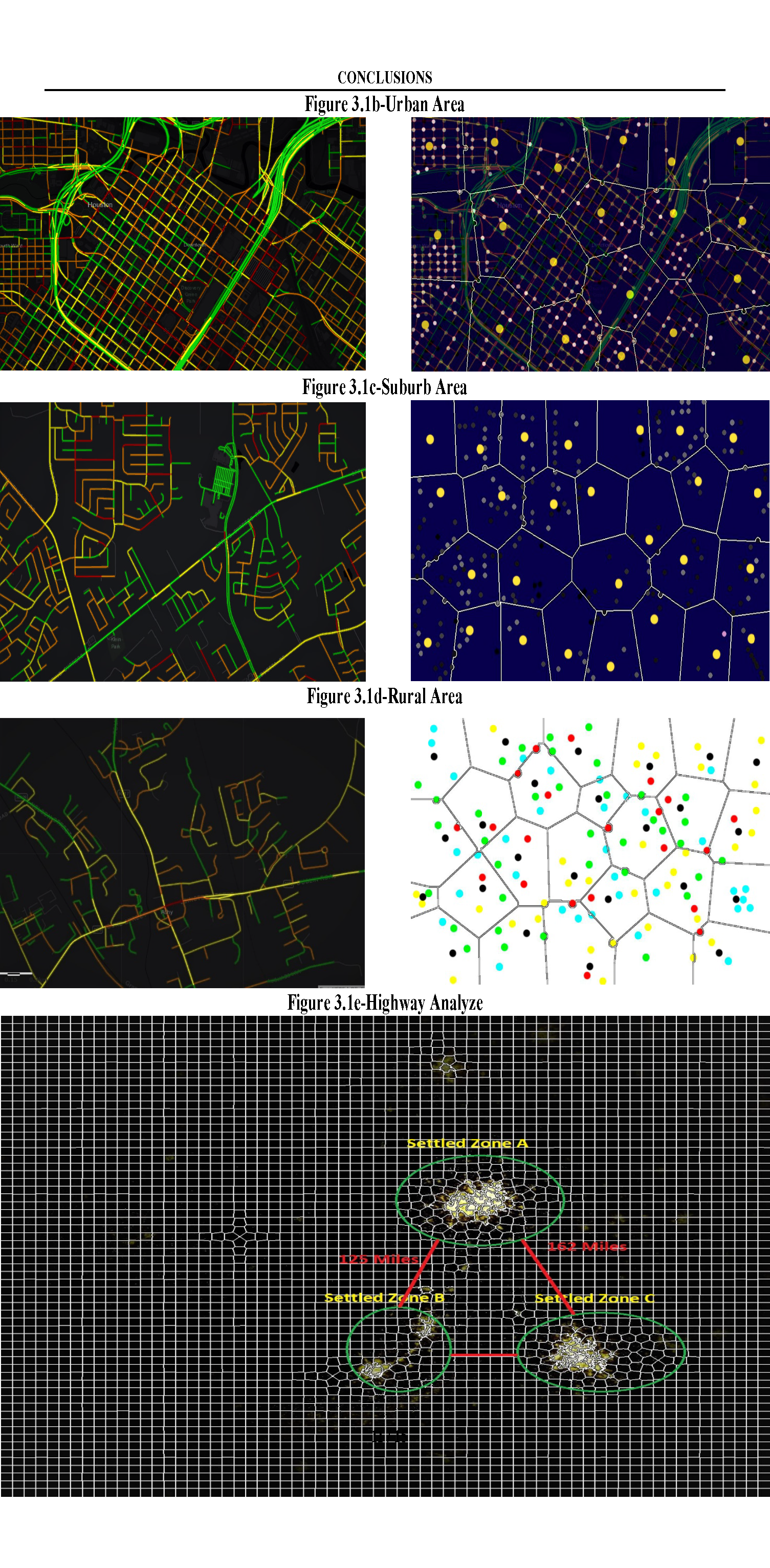
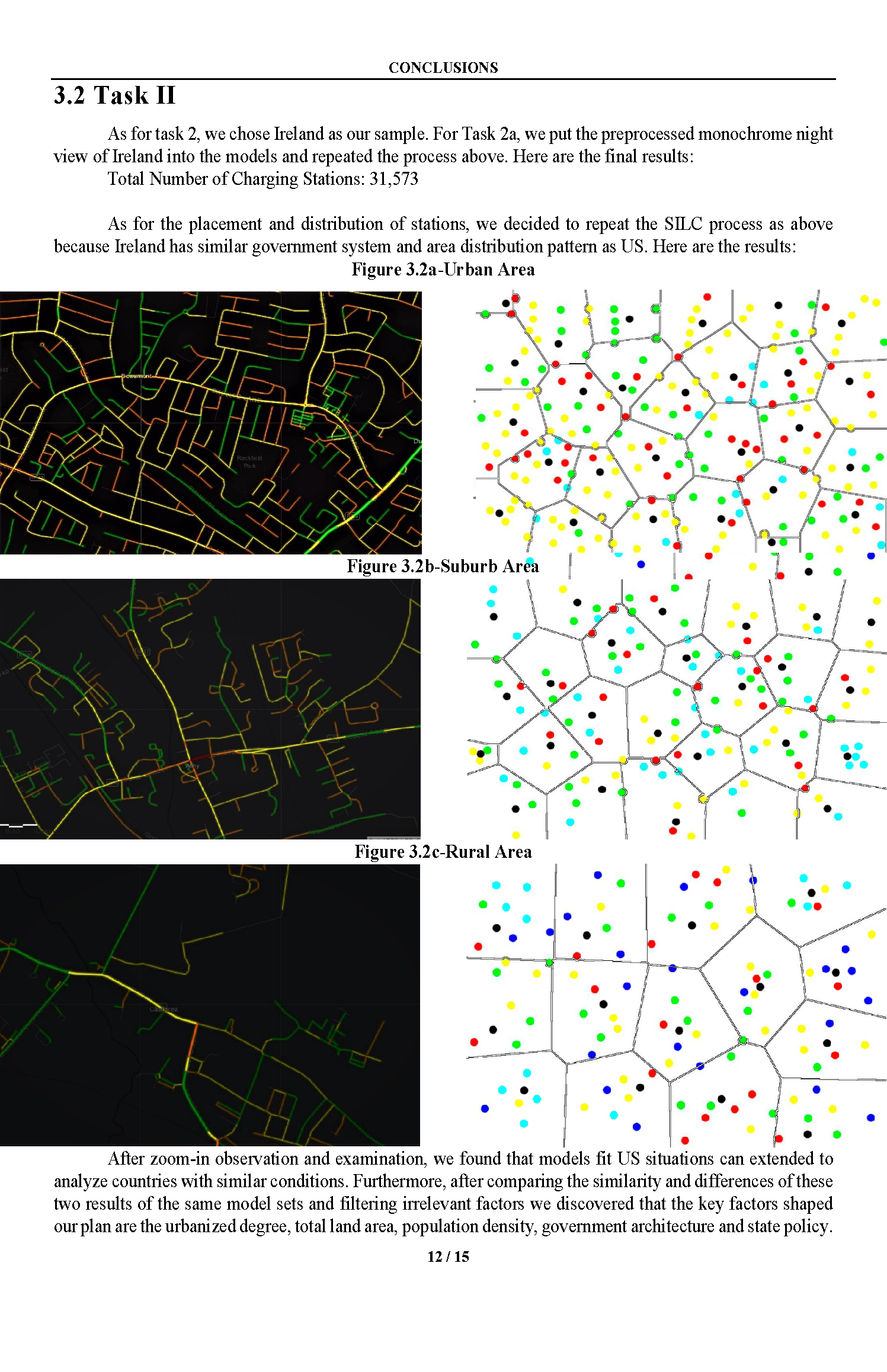
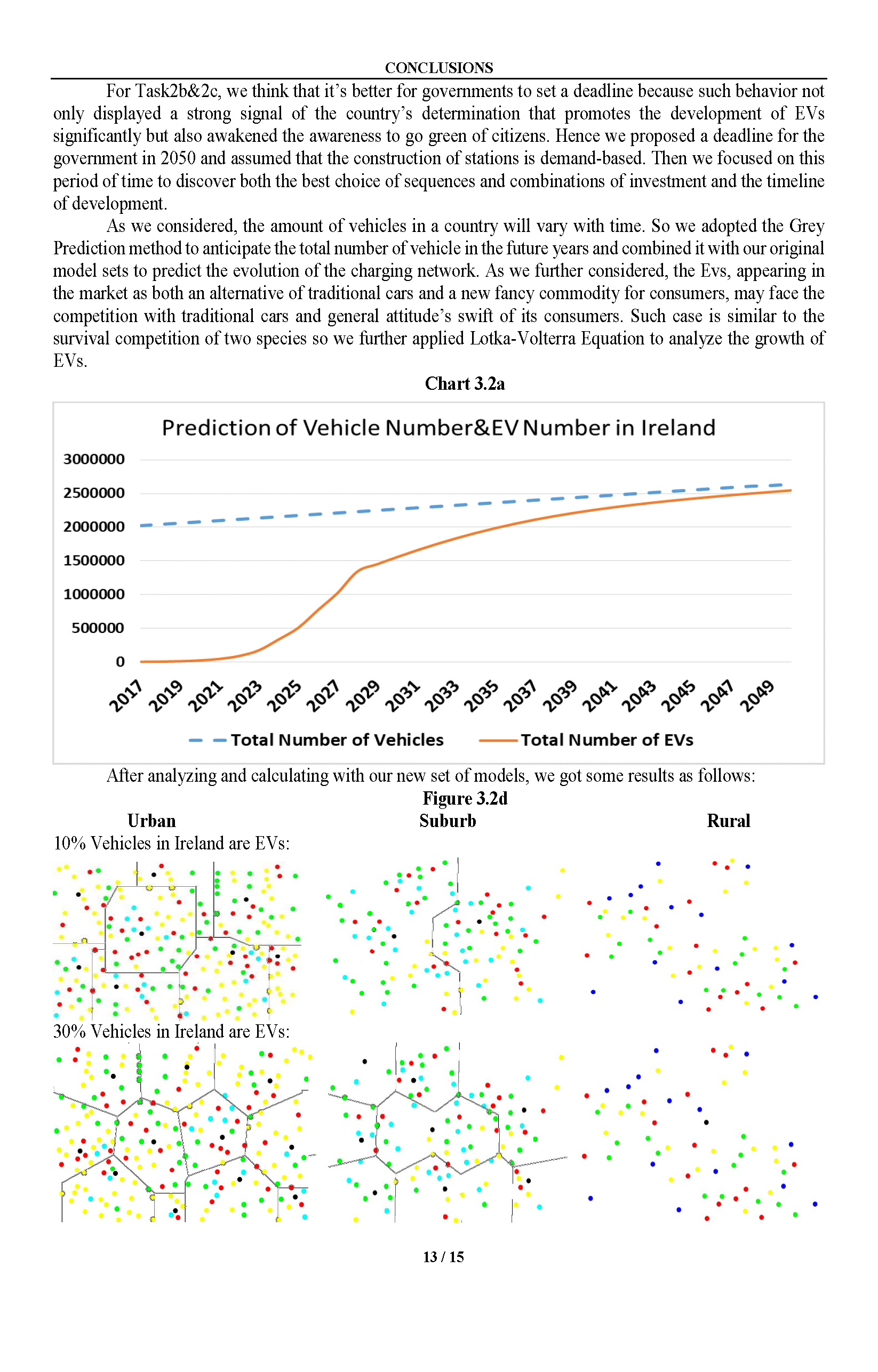
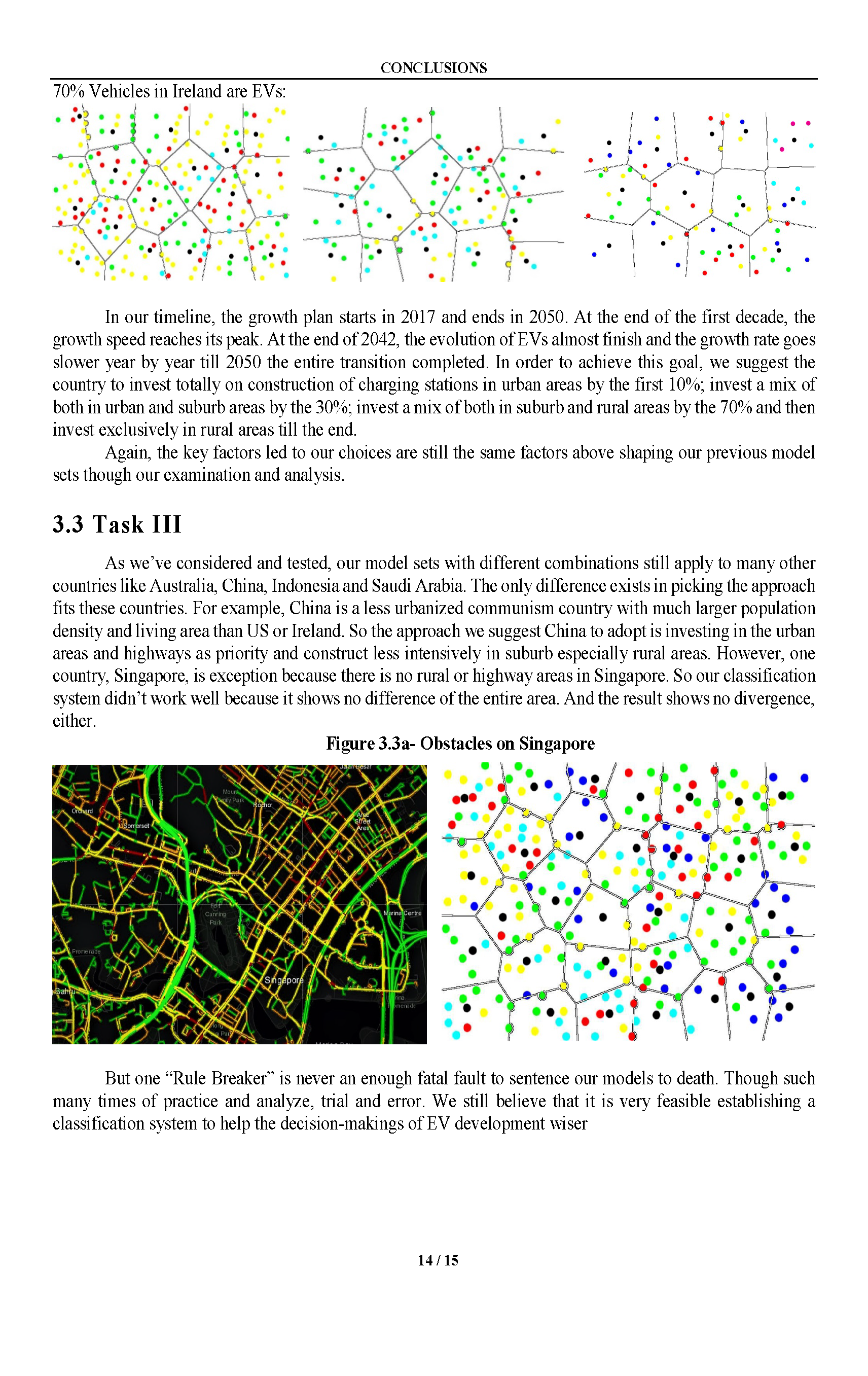
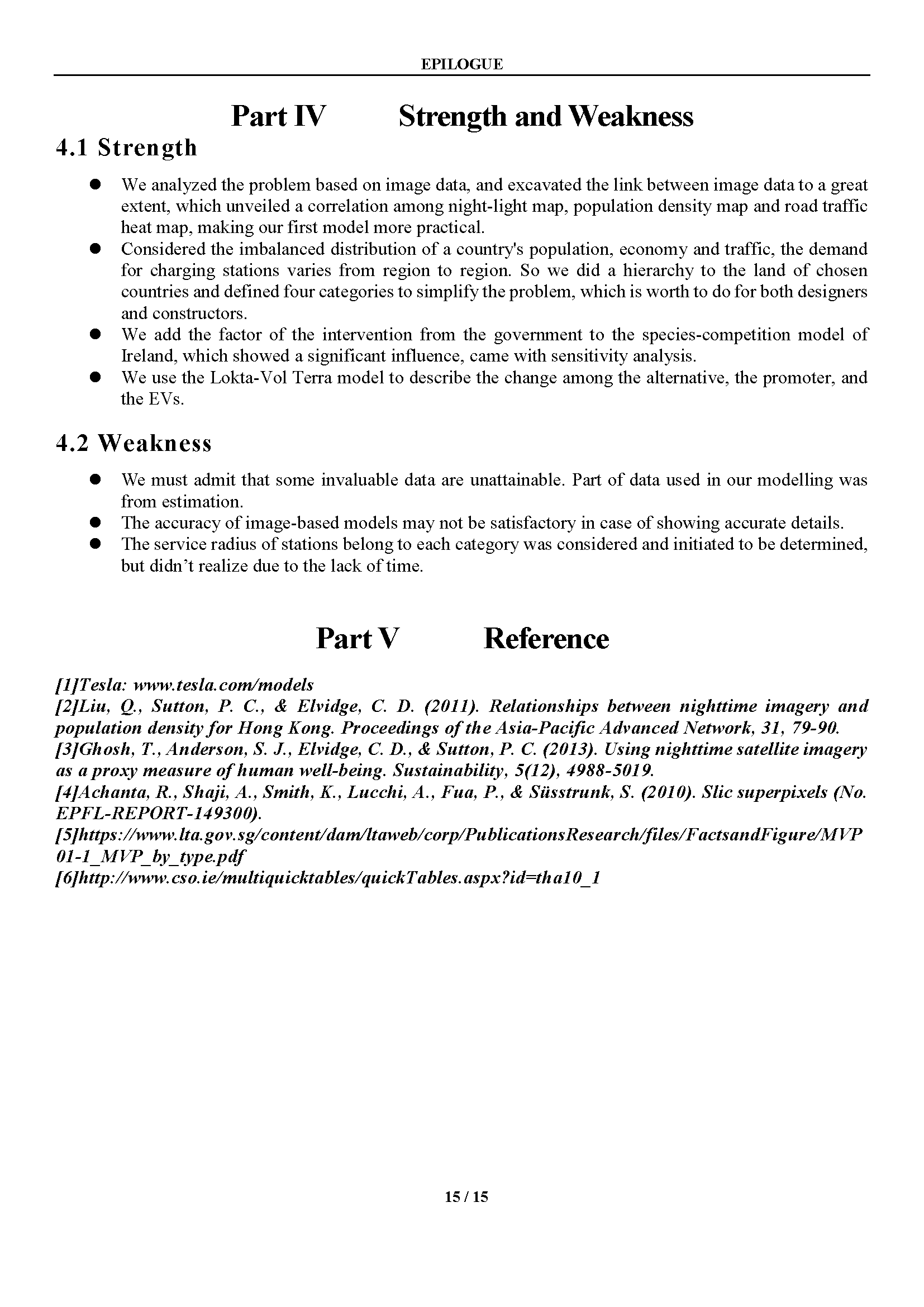